Addressing AI’s Adoption Gap Problem in Healthcare
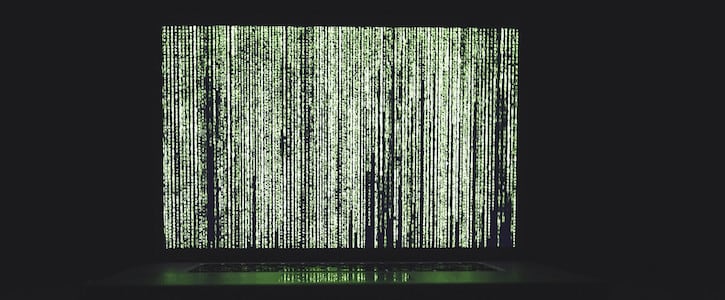
The steady drumbeat of optimistic forecasts for artificial intelligence (AI) adoption across sectors has continued unabated this year. Analyst firm Frost & Sullivan fired the latest round with a report predicting that AI and cognitive computing will save $150 billion for the healthcare industry by 2025. The report checks off all the usual suspects that will contribute to the savings: disease prediction, personalization of care pathways, claims processing and supply chain operations. Then comes the letdown: AI adoption in healthcare been slow due to “strategic and technological” challenges, and only 15-20 percent of end users have been actively using AI to drive real change in the way healthcare is delivered.
>> READ: The Rising Clamor for Explainable AI
The stubborn resistance to AI adoption in all industries is now a reality that everyone is coming to terms with. Consulting firm McKinsey says that only a small fraction of the technology’s potential value has been realized across all sectors — as little as 10 percent in some industries. To make sure that these downbeat forecasts don’t turn off potential clients, McKinsey helpfully points out that there is a wide and growing performance gap between high and low adopters of AI, implying that the laggards need to catch up.
Something is worrisome about the whole picture.
First, let me address the vendor side of the picture and the current state of AI offerings. Unbridled marketing hype and inflated claims of “AI-enabled” solutions have confused many potential buyers and left others deeply skeptical. Black-box algorithms have created an “explainable AI” problem that I discussed in an earlier column here. Health systems that made early bets on promising technologies have pulled back sharply in the face of tepid, and often misleading, results. Healthcare is nothing if not conservative, and for two good reasons, if not more: Patient safety is more important than anything else, and there isn’t much spare cash sitting around for expensive, cutting-edge technology experiments. Most health systems want results now, preferably yesterday.
I am not suggesting that AI has no takers or that there is no real progress. Our research indicates that many academic medical centers (AMCs) and some of our public health institutions, including the National Institutes of Health and the Centers for Disease Control and Prevention, are quietly investing in advanced analytics programs. Grant funding for AI projects is sustaining long-term research focused on finding cures for diseases. Public-private sector partnership is thriving, as are research partnerships between AMCs and big technology firms.
The story with commercial health systems is entirely different. Caught in the pincer grip of a rapidly changing reimbursement environment and an increasingly demanding healthcare consumer (not to mention serious nontraditional competitors waiting around the corner), these health systems are struggling to come to terms with changes in the marketplace and their own business and operating models.
Here are three key challenges for health systems looking to advance the ball with AI programs today.
1. Finding an organization model for the future.
In an insightful piece, strategy guru Michael Porter of Harvard Business School and Thomas Lee of Press Ganey argue that healthcare has an org model problem, similar to that faced by industrial conglomerates in the mid-20th century. They argue that the way health systems are organized today, around physicians, is archaic. While the model works well in a fee-for-service reimbursement era, it will no longer work in a value-based care era with heightened consumerism.
The problem has its echoes in analytics efforts as well. The current org model makes health systems victims of data silos that refuse to come together, and when they do, houses them in standalone analytics divisions that very often do not report into the CIO of the organization. With multiple points of ownership for data assets, data sciences talent and computing infrastructure, even the most basic analytics programs struggle for traction at the enterprise level. AI requires an entirely different level of commitment at an organizational level and the establishment of specialist cross-functional teams with a common leadership.
2. Achieving scale economies.
AI requires vast amounts of data (and not insignificant amounts of financial commitments). AI programs may fail to take off simply because there aren’t enough data to work with to demonstrate real benefits. The widely publicized woes of IBM Watson Health pertain partly to this problem.
One way to address the issue of scale economies is to develop consortiums that can pool data for research and benefit from the results. The recent announcement by 41 health systems, led by Geisinger, Intermountain and others, to collaborate on reducing diagnostic errors could be an interesting model for the future. Similar initiatives are already in flight; Apple’s collaboration with several health systems across the country will deliver personalized health insights through the iPhone. An innovation collaboration by 17 health systems to improve Medicaid outcomes, led by Andy Slavitt, former Centers for Medicare & Medicaid Services administrator, and AVIA, is another.
3. Addressing stranded costs.
Health systems are at a difficult crossroads today, forced to choose between continuing with the (currently profitable) status quo versus positioning for the future. Most health systems have made significant investments in their core systems of record, such as electronic health records (EHR), and are under pressure to optimize their use of the systems for improved returns.
But for programs such as advanced analytics and AI, EHR systems are not necessarily the right choice for advanced analytics. Other challenges may include partly depreciated investments in expensive data center environments built for the deployment of on-premise technologies in an era when the most advanced technologies are available almost exclusively on cloud infrastructure. The appetite for expensive AI programs with limited visibility to returns is even less when there is fierce competition among competing internal initiatives. Progressively moving workloads to the cloud and migrating on-premise software licenses to the cloud are some of the options available to continue to pursue innovation with AI.
The AI Takeaway
Most health systems realize that a failure to adopt emerging technologies such as AI could doom them in the future as performance gaps increase not just among themselves, but more crucially between incumbents and the emerging crop of big tech firms such as Apple and Amazon, who are aggressively moving into traditional care delivery with owned and operated primary care clinics. For now, health systems have a choice: pursue competitive advantage and sustainability through advanced technology investments such as AI or risk becoming frogs in boiling water. I know the answer: Show some money — now.
Originally published on idigitalhealth.com