The Rising Clamor for Explainable AI
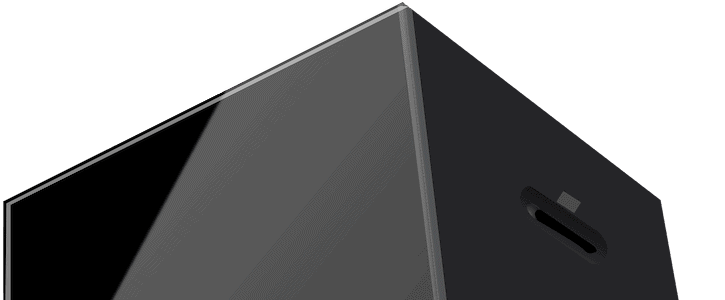
There’s no doubting the enthusiasm behind artificial intelligence- (AI) powered healthcare. A recent Accenture survey found that 53 percent of healthcare executives expect to invest in AI, with 86 percent noting that their organizations are capitalizing on data to drive automated decision making at an “unprecedented scale.”
However, this survey also demonstrates the vulnerability that these executives are feeling. A stark majority has not yet invested in the ability to validate data sources across the most mission-critical systems, and 24 percent said they have already fallen victim to “adversarial” AI behaviors, including falsified location data and bot fraud. This raises alarm bells about the potential for abuse with AI in areas such as unnecessary profiling of patients or actual errors in diagnoses and treatment of medical conditions.
There is a growing realization that potential users of these advanced tools for clinical decisions want to see what’s inside the “black box” of AI to ensure that the technology is advancing care, instead of sowing mistrust on the part of patients and providers. In short, AI needs to explain itself.
Why Make AI Explainable?
The clamor for transparency into how an AI algorithm works is growing in the healthcare and patient communities. One concern relates to the potential for bias within the algorithm. As an example, bias can take the form of improper weighting of one demographic group over another, which could result in racial profiling and even push an inappropriate treatment option on the wrong group of patients.
Sheila Colclasure, chief data ethics officer at Acxiom, echoed this sentiment with me during a recent podcast, as she explained why her organization now focuses on the idea of data ethics. “Data ethics is not just about minimum compliance but also about being ‘just’ and ‘fair,’” she said. It is essential to mandate data ethics at the engineering layer in today’s cloud-based systems, she added. In the context of AI, this translates to ethics and transparency at the algorithmic layer, providing users of AI-based technologies with visibility into how such algorithms work.
Take the example of neural networks, which are systems that can process thousands or millions of binary data points to mimic human decision making and identify health issues based on patient data. This approach has shown promising results in imaging data for the detection of diabetic retinopathy. However, there is a recognition that unless a clinician knows how a neural network is arriving at a decision (such as predicting diabetic retinopathy through the analysis of images), any recommendations to the clinician will not be trusted. Without “explainability,” much of the potential of AI might be lost, as clinicians continue to rely on traditional methods of diagnosis and treatment.
A related rule of thumb: The higher the medical risk and complexity involved in a clinical decision, the less likely it is that experienced clinicians will defer to an algorithm to decide on their behalf. The widely publicized challenges of IBM’s Watson Health platform in providing treatment recommendations for oncology is directly related to the lack of transparency around their cognitive techniques embedded in the platform.
The push for greater transparency is needed more than ever with uncertainty also building around regulatory and legal issues surrounding AI and other technologies used in healthcare. A recent look at automated systems used to perform surgery raised questions on just who is liable if these systems are not under the guidance of a physician and they make a mistake. Is it the physician using the device, or the manufacturer the one who should be held accountable? Moreover, how should regulations be crafted to address just such an issue?
Regulating (and Self-Regulating) AI in Software
The FDA is attempting to create a new approach that is voluntary for companies developing software and medical devices. The goal is to protect the healthy growth of technologies such as AI and keep pace with fast, iterative designs, while still providing necessary oversight in the interest of consumer protection and patient safety. With the FDA’s recent announcements, this takes the form of a precertification program that utilizes a “trust-based approach to regulation for those vendors that have shown that they embrace a culture of safety and accountability.”
>> LISTEN: Finding Fault When AI Kills
While still under development, so far the FDA is considering 12 categories to evaluate vendors including leadership, transparency, people, infrastructure and work environment, risk management, configuration management/change control, and so on. The plan is to tie key performance indicators to these categories to keep watch on an organization once they have been approved, but individual products would not need to be regulated.
Beyond the FDA, industry organizations have started taking the lead on defining guard rails for the use of AI in healthcare technology. The American Medical Association (AMA) passed a policy on AI (its first) that starts to address the technology as “augmented intelligence.” The idea is to shift thinking on how to develop AI into something that enhances the clinical decision making of physicians but does not replace them. According to the AMA’s May report, “Artificial intelligence constitutes a host of computational methods that produce systems that perform tasks normally requiring human intelligence. However, in healthcare, a more appropriate term is ‘augmented intelligence,’ reflecting the enhanced capabilities of human clinical decision making when coupled with these computational methods and systems.”
Whether it’s new terminology pushing for a rethink of what the goals of AI should be, a new regulatory framework, or new technology to make AI explainable to those who depend on it most, the reality is that transparent AI is the key to driving adoption levels higher. Moreover, it’s also critical if we want to protect public perceptions around AI, especially considering how much we’re going to need the technology if we’re going to fix our broken healthcare system. We are still in the early stages of opening up the algorithms for scrutiny—partly due to concerns around proprietary knowledge and intellectual property that most firms are reluctant to share. The growing clamor for explainable AI may be the best thing to happen to purveyors of AI-based technologies who face an increasing credibility problem.
Originally published on INSIDEDigitalHealth.com